Managing Python Environments in 2022 (for the 99%)
April 25, 2022 •TL;DR: Install mambaforge, use mamba to install packages, and pin direct dependencies inside your environment.yml.
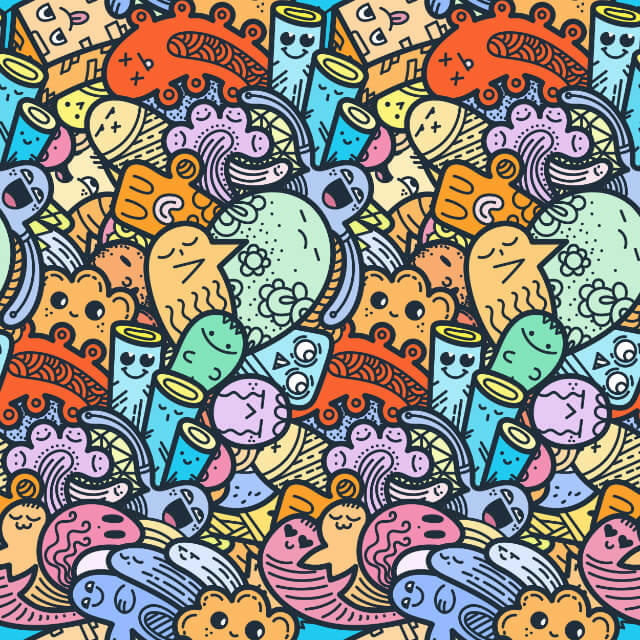
Pip, venv, virtualenv, pyenv, pipenv, micropipenv, pip-tools, conda, miniconda, mamba, micromamba, poetry, hatch, pdm, pyflow 🤯 These days even the most senior Python developer is confused about all the options to manage environments.
Instead of untangling the whole convolutated mess that is the Python environment management ecosystem by means of a thorough explanation, I decided to go a different route, not unlike another Alexander did in the past:
The Legend of the Gordian Knot
In 333 B.C. Alexander the Great encountered an oxcart tied to a post with a complicated knot that seemed to have no beginning and no end. Legend had it that whoever solved the problem of the knot would rule all of Asia. As his goal was to rule the world, this was a challenge he could not pass up. He studied the knot for several days and could find no way to untie it. Asking “Does it matter how I solve the problem?” he drew his sword and cut the knot, exposing the ends required to untie the knot.
That night there was a great storm and the prophets took it as a sign that Zeus was pleased. Alexander went on to rule not only Asia, but much of the world. (source)
So in true Alexandrian fashion, I’ll cut through the mess and
- (1) flat-out propose that you should use conda (Mambaforge, to be precise);
- (2) give you best practices for managing Python environments with conda;
- (3) explain how we ended up with all these tools, ELI5 style.
If you’re unsure, use Mambaforge
Some people get really worked up about The Right Way to Manage Environments. If you are happy with your current workflow, by all means, stick to it. But differences aren’t so pronounced as people make them out to be, often due to various misconceptions. You can make most of the tools work for most cases, with most differences boiling down to matters of personal opinion.
For almost everyone else, for the 99% developers, my suggestion is:
Install mambaforge, use
mamba
to install packages, and pin direct dependencies inside yourenvironment.yml
.
Mambaforge is a so-called “conda-forge distribution,” combining mamba (a drop-in replacement for conda) with conda-forge. This gives you access to the following things:
- a minimal Python installation (mambaforge)
- a virtual environment manager (
mamba env
) - a package manager (
mamba install
) - a fast dependency solver (libmamba, which is much faster than conda’s solver and will eventually become the default)
- a vast ecosystem of precompiled packages (conda-forge)
- a big community of developers around the project (less technical risk!)
- cross-platform support (Linux/Mac/Windows)
- an emphasis on support for various CPU architectures (x86_64, ppc64le, and aarch64, including Apple M1)
- I repeat: first-class support for Apple M1 binaries! :-)
Possible Objections
But Alex, I’m managing dependencies for my Docker image; installing mambaforge will blow up my image size!
No, you’re doing it wrong: use multi-stage builds.
But Alex, I’m developing a Python library that I want to distribute through pypi, and poetry is much better at that.
If you’re creating a (pypi) package, then you’ll want to add something like poetry or hatch to your stack. So if that’s your main concern and you don’t need any of the benefits mambaforge gives you, then it might make sense to ditch mamba and use poetry for everything. Otherwise, I’d stick to my recommendation and just add poetry for its packaging capabilities on top of mambaforge, which will take care of both package and environment management.
Conda Best Practices
There are a few things you should know for managing your environment with conda (mamba) on a day-to-day basis, so keep in mind the following best practices:
- Install everything you need using
conda install
/mamba install
. - If (and only if) the package isn’t available through conda-forge (or any other channel), fall back to using
pip install
(this could potentially lead to conflicting dependencies, but it’s rare enough to not worry about it too much). - When working on a Python package, pin (at least all direct) dependencies in your
environment.yml
. Be more strict with version numbers if it’s an app, less strict if it’s a library. - If you need to get real serious about pinning dependencies, read up on conda-lock.
- Use the
conda-forge
channel; don’t use thedefaults
channel (due to its licensing issues). Miniforge/mambaforge distributions come with this change pre-configured. You can also set your channels manually inside your~/.condarc
file:channels: - conda-forge
- Run the installer in silent mode (
-b -p <installation_path>
), so you don’t have to be prompted for anything. Silent mode installation is especially useful for bootstrapping your newly launched cloud instances, for example. Here’s how you can silently install mambaforge:export MAMBAFORGE_DIST=Mambaforge-$(uname)-$(uname -m).sh wget "https://github.com/conda-forge/miniforge/releases/latest/download/$MAMBAFORGE_DIST" bash $MAMBAFORGE_DIST -b -p ~/mambaforge && rm $MAMBAFORGE_DIST ~/mambaforge/bin/conda init bash # restart shell after this
- Bonus tip: To bootstrap my base environment with all the libraries I frequently use for prototyping (black, jupyterlab, transformers, etc.), I keep a yml file specifying my base environment in my home dir and then update according to the spec using:
Caution: If you do this, you’ll have to promise to create a project-specific environment and refactor all your notebooks into proper packages at a later stage!mamba env update --file ~/.python-environment.yml
An imaginary tale of how we got ourselves into this
To understand how we ended up with so many tools, I think it helps to think in terms of successive improvements on the status quo. Now I don’t know about the actual genesis, so I’m taking some poetic license to sacrifice historical accuracy for the sake of understanding, but you could imagine the progression of tools as follows:
Python is cool, but I need external libraries → pip
pip is cool, but I need to have different versions of the same library installed at the same time → venv, virtualenv
virtualenv is cool, but I need to have different versions of python installed at the same time → pyenv
virtualenv and pyenv are cool, can I have both? -> pyenv-virtualenv
Virtual environments are cool, but I’d like to pin my dependencies for better reproducibility →
pip freeze > requirements.txt
Pinning dependencies is cool, but I want to separate direct dependencies from transitive dependencies → pip-compile, conda-lock
Virtual environments are cool, but they should work on a per-project basis → pipenv
pipenv is cool, but I want to use it in a lightweight container → micropipenv
pipenv is cool, but I focus more on library development than on app development → poetry
All these things are cool, but my project relies on non-Python packages → conda
conda is cool, but the dependency resolution is really slow → mamba
conda is cool, but users should be able to contribute packages to the ecosystem → conda-forge
conda and conda-forge are really cool, they should come prepackaged with a little installer → miniforge
miniforge is cool, but I prefer to use mamba instead of conda → mambaforge
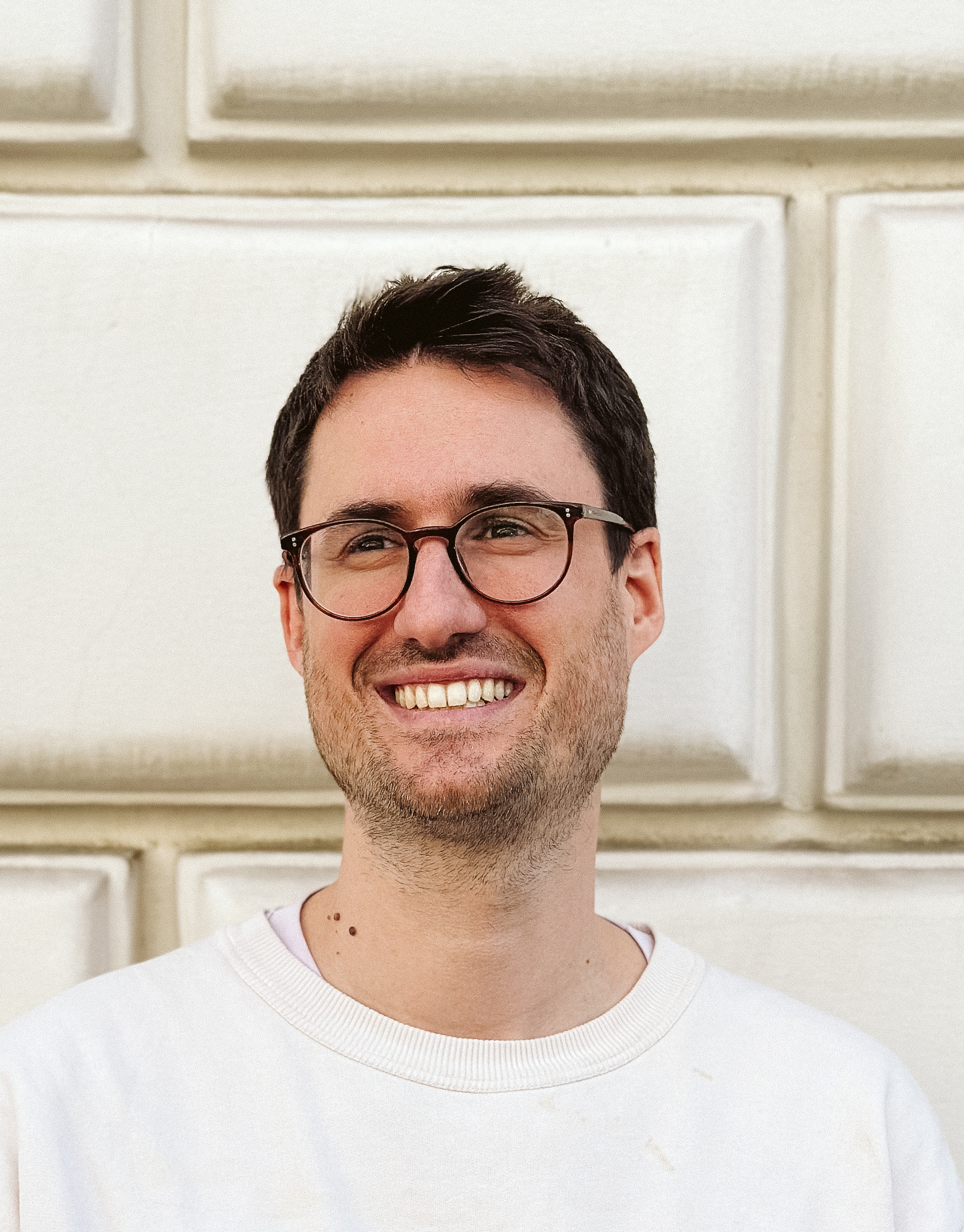
Alexander Seifert
Hi, I'm Alex and I write this blog. Here you'll find articles and tutorials mostly about Natural Language Processing and related areas.
Follow me on Twitter for updates or contact me.