A Gentle Introduction to AI: Resources for Decision-Makers
March 14, 2022 â˘A list of the most valuable resources for decision-makers to learn just enough about AI to get going.
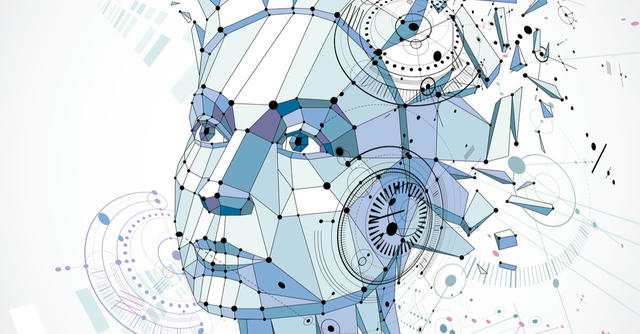
Another day, another news article about your competitor launching a hot new feature built with AIâ˘. AI winter is over, and decision-makers are eager to put these promising new technologies to use. While the potential gains can be huge, Machine Learning projects are inherently risky and vastly more complex than traditional software engineering projects. Many companies find out the hard way, as illustrated by the fact that Gartnerâs estimate that 85% of AI projects fail is still being cited all the time.
One of the most critical steps that business leaders can take to avoid the fate of doomed projects and disenchanted teams is to identify the right business problem to solve. Unfortunately, we canât trust our intuitions about the capabilities and limitations of AI. This is known as Moravecâs paradox, after the Austrian-born Canadian roboticist Hans Moravec, who described this counterintuitive fact in 1988. This paragraph taken from Steven Pinkerâs book The Language Instinct, published in 1994, is still valid after another three decades of AI research:
The main lesson of thirty-five years of AI research is that the hard problems are easy and the easy problems are hard. The mental abilities of a four-year-old that we take for granted â recognizing a face, lifting a pencil, walking across a room, answering a question â in fact solve some of the hardest engineering problems ever conceived [âŚ] As the new generation of intelligent devices appears, it will be the stock analysts and petrochemical engineers and parole board members who are in danger of being replaced by machines. The gardeners, receptionists, and cooks are secure in their jobs for decades to come.
The fundamental insight to resolve this apparent paradox, I believe, is to understand that the metaphor of âartificial intelligenceâ is just thatâa metaphor; and since artificial intelligence is vastly different from human intelligence, our intuitions about one donât readily transfer to the other. But without proper intuitions, picking the right problem to solve is incredibly difficult. Thatâs why Iâve been thinking a lot about how to explain the technology to non-technical people and how to instill good intuitions about use-cases suitable for Machine Learning.
Over the years, I have read thousands of articles and blogs, taken dozens of courses, and watched endless hours of videos about AI. Most of the content aimed at a non-technical audience is full of hype, grave inaccuracies, and hidden marketing messages. If youâre not an expert, separating the wheat from the chaff is nearly impossible. Below, I have selected only the best, most valuable resources for decision-makers to learn just enough about AI to get going.
Courses
To get started, I suggest you watch at least Part 1 and 2 of Cassie Kozyrkowâs Making Friends with Machine Learning, a series of talks where you will acquire an excellent high-level overview of AI/ML. The first two parts combined last for three hours, are highly engaging, entertaining, and non-technical. Itâs an initially Google-internal course given by Googleâs Head of Decision Science, Cassie Kozyrkow, âcreated to inspire beginners and amuse experts.â
The entire 6.5 hours course has four parts:
- Part 1: Introduction to ML
- Part 2: Life of a Machine Learning Project
- Part 3: AI from Prototype to Production
- Part 4: Opening the Black Box
I also highly recommend the following mini-course (4.5 hours of videos) called AI for Everyone. It is led by Andrew Ng, a Machine Learning pioneer whoâs the co-founder of Coursera and an Adjunct Professor at Stanford University. Specifically, youâll solidify your understanding of:
- What AI can and cannot do
- How to spot opportunities to apply AI to problems in your organization
- What it feels like to build machine learning and data science projects
- How to work with an AI team and build an AI strategy in your company
Blog posts
If youâd rather read some well-written pieces than watch videos (or do both), then this section is for you. Without exception, all of the following articles were written by Cassie Kozyrkow, whose content on ML I canât recommend highly enough. Donât get put off by the somewhat clickbaity titles. I promise that reading those blog posts will be worth your time.
In the first article that I want to highlight, youâll learn why the right first step is to focus on outputs and objectives:
Imagine that this ML/AI system is already operating perfectly. Ask yourself what you would like it to produce when it does the next task. Donât worry how it does it. Imagine that it works already and it is solving some need your business has. [âŚ]
A kitchen analogy comes in handy here. If youâre running a restaurant (as opposed to an appliance factory or food science lab), why would you think about buying â or, worse, inventing â a pizza oven before youâve even considered whether adding pizza to your menu makes sense? That sounds like the rookie mistake of someone who doesnât know what business theyâre in. Instead, start with what your customers want and what food quality youâre willing to settle for. [âŚ]
Applied AI requires you to have a very clear vision of what your model needs to grow up into (and why), then you have to train it towards that. If you donât know what you want, head to the font of inspiration instead: analytics.
Cassie follows with another blog post describing how you make that first step. (She also has a much more extensive version of that article called The Ultimate Guide to Starting AI, where she lists 22 questions you should think about before you start an AI project.) The essential steps are to:
- Figure out whoâs in charge
- Identify the use case
- Do some reality checks
- Craft a performance metric wisely
- Set testing criteria to overcome human biases
(If you are a checklist-person and want to know how to avoid the biggest traps, then she also has you covered: Is your AI project a nonstarter?)
The last post I want to highlight is the following, where Cassie presents a super-useful trick for coming up with problems suitable for machine learning:
I want you to imagine for a moment that there is no machine learning. Itâs all a hoax; thereâs just an island in the middle of the ocean somewhere with a bunch of my friends behind computer screens pretending to be an AI. When you send them an input, they quickly send back a decision. For example, you send that cat photo and they sneakily type âcatâ for you so it looks like cool machine learning.
Hereâs the fun part. Iâm going to let you borrow my island to use for your business. Free of charge. Thereâs only catch: you canât write them any instructions â my friends are weird like that. You can only teach them with examples, but theyâre fast and numerous. Itâs a pain to spend time teaching them if you have a one-off task, so focus instead on the repetitive drudgery youâd like to cut out of your life. What would you use this island for?
Going Deeper
If you want to go deeper, start with Cassie Kozyrkowâs blogpost, which I already shared above, and then simply follow all the links that pique your interest. Her writing is that good.
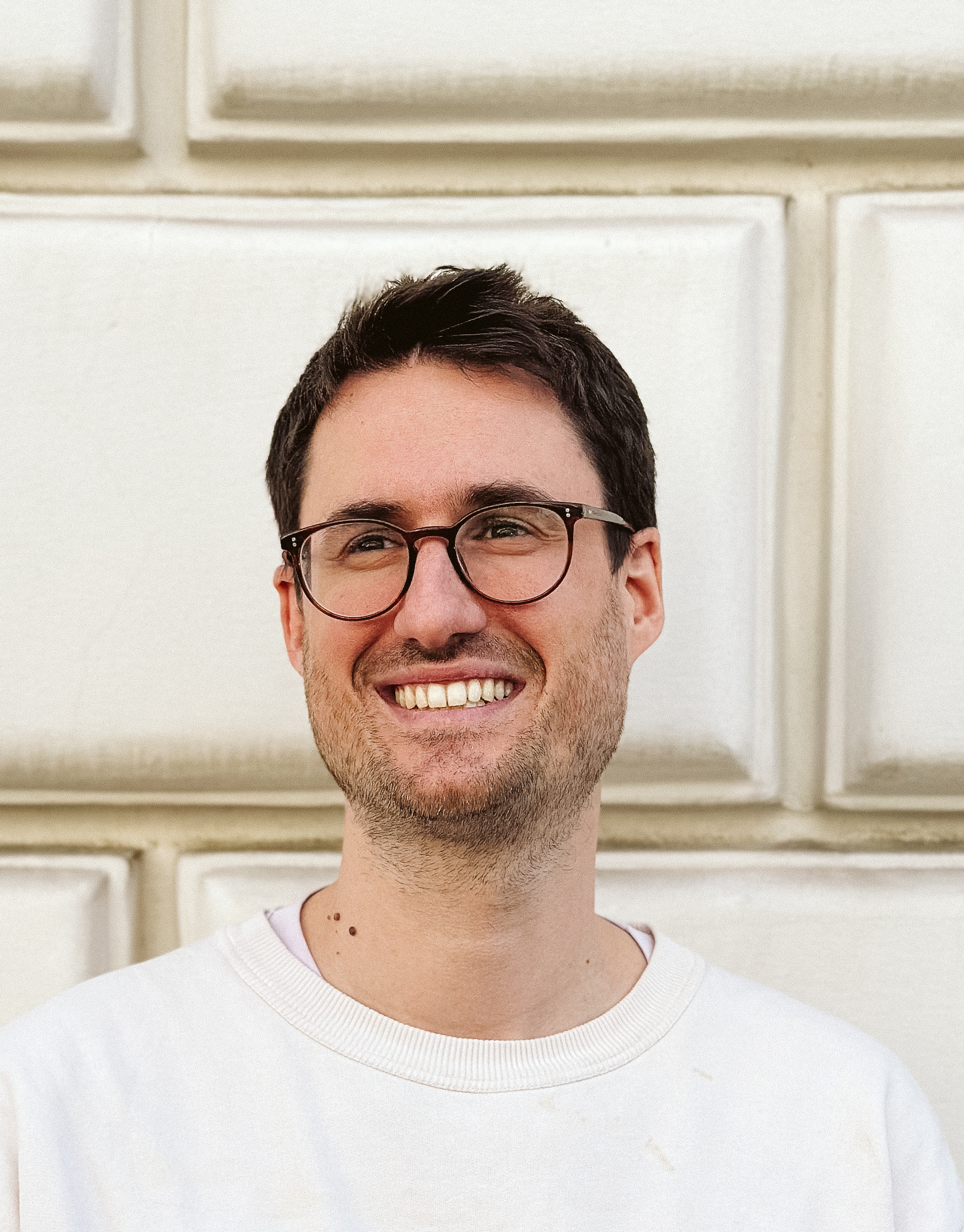
Alexander Seifert
Hi, I'm Alex and I write this blog. Here you'll find articles and tutorials mostly about Natural Language Processing and related areas.
Follow me on Twitter for updates or contact me.